Building a Working Knowledge of Salesforce Service Cloud Einstein
The author of this article is EPAM Associate Director Salesforce Practice Jolene White.
Introduction
In the recently published Salesforce’s State of Service 5th Edition, it highlights a shift in focus to efficiency and case deflection, along with all of the other standard service KPIs. Service Cloud Einstein combines powerful features to help automate a service organization, by deflecting cases and reducing agent busy work. By building a working knowledge of Service Cloud Einstein, you can kick start an assessment of which features are best suited for your organization.
As much as we would all like to ‘just turn on’, and see it magically deliver results, it is not quite that easy. But with some planning and analysis, you will be wowing your service agents in no time. Please refer to the tables, for an overview of more specific details alluded to below.
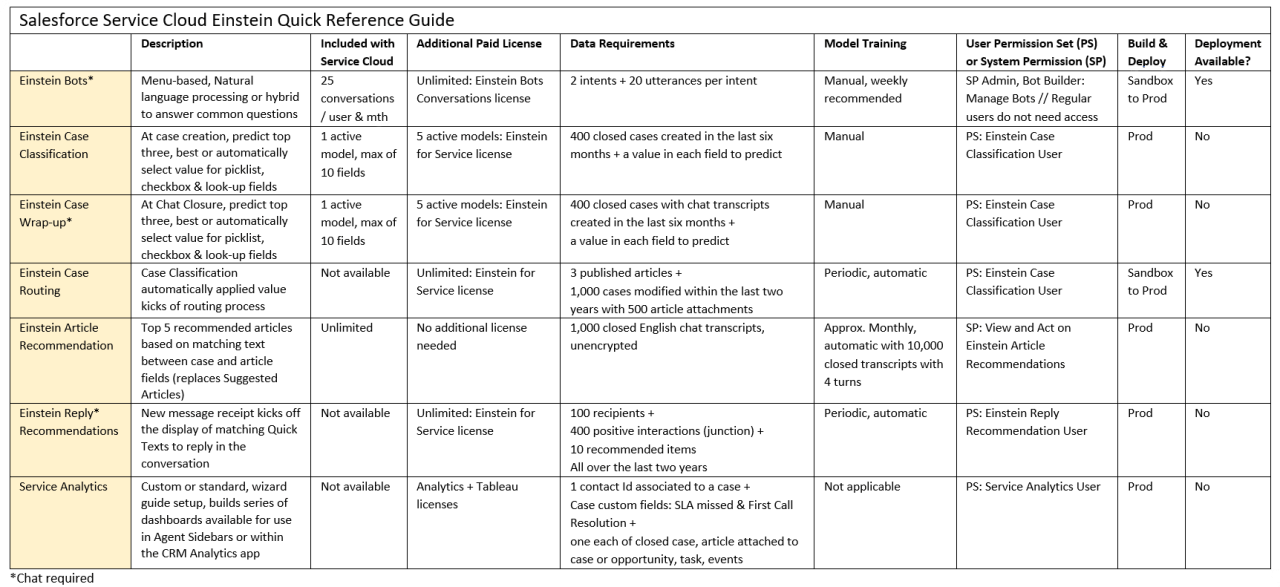
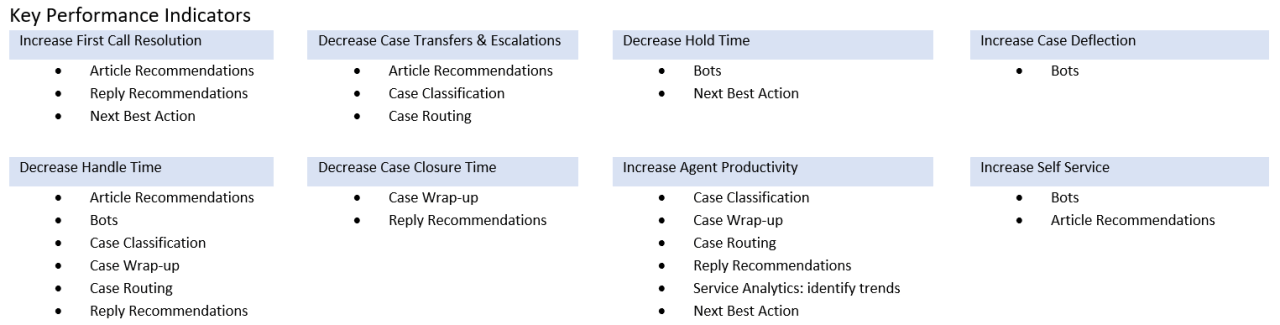
Service Cloud Einstein features
The core features are:
-
Einstein Bots*
-
Einstein Case Classification
-
Einstein Case Wrap-up*
-
Einstein Case Routing
-
Einstein Article Recommendations (note: this replaces Suggested Articles)
-
Einstein Reply Recommendation*
-
Service Analytics
*Features require Salesforce Chat implementation.
Supporting Products, not technically within Service Cloud Einstein, but important to an overall solution:
-
Einstein Next Best Action
-
Einstein Recommendation Builder
Most of the features require additional licensing, however, several of the features are available for use with a non-chat, Service Cloud implementation:
-
Einstein Case Classification
-
Einstein Article Recommendations
-
Next Best Action with Einstein Recommendation Builder
How much data do you need? How does the model get trained after initial launch?
As part of the setup process, Salesforce will scan your environment to verify if you do or do not have enough data, and let you know the outcome.bAfter the initial setup, it could take up to 24 hours for the model to be trained and tested.
It varies between features if the re-training will be manual or automatically done for you. Regardless of how the model is continually updated, it should be monitored for relevancy and accuracy, so your service team achieves the greatest benefits.
How do the features get built, tested and deployed?
Because Einstein is finding patterns in an environment’s specific data set, most of the features need to be built directly in production. However, you can take a sandbox and do a test run of the feature’s build, but you can’t move it to production with any available method. You will have to rebuild it.
To prevent your production users from seeing the new features, only grant them the permission sets and visibility to the component when you are ready.
How is the impact of Service Cloud Einstein measured?
Monitoring the models and impact of Service Cloud Einstein features is available four ways:
-
Setup
-
Standard Reports / Dashboards
-
Service Analytics + Tableau
-
App Exchange Dashboard/Reports
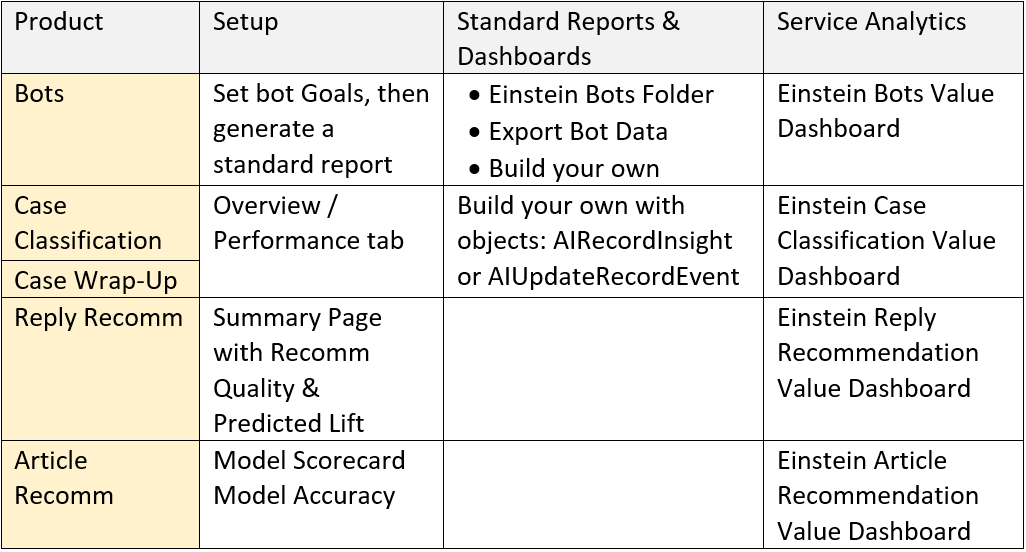
How do you get started?
1. Start small with features already included in your Service Cloud license.
2. Identify what KPI you want to impact, so you select the correct tool for the problem.
3. Commit to doing the best implementation of the one feature you select, before moving onto the next feature.
4. Review and clean-up existing case and article data, so Einstein can provide the best insights.
5. Engage your end-users in the entire process, so you create a working partnership and natural feedback loop.
6. Create a separate team to review, write, and build your articles, if you don’t currently have any.
7. Assign a small team who will continue to monitor and iterate on the models once established, so you get the most out of your implementation. Make sure their time is committed every month to this activity, so end-user feedback and the models are incrementally improved. The implementation and ongoing maintenance of these features is never truly ‘done’. This type of project reaches milestones of accuracy and impact on your KPIs.
8. Plan on celebrating success throughout the process, with the team and end-users, who are all needed to make it possible.