Career Switch to Data Science: Unlocking New Opportunities
The author of this article is tech expert Pieter Murphy.
A mid-career switch is never an easy choice. It is hard to leave your current work-life routine, nor to forgo any career investments up to this point. There are also financial considerations, skills gaps, and worries about how you fit into a new company culture.
But a change of scene might be the exact right option for you—especially a switch into data science. Data science has become one of the most attractive career paths as enterprises place greater value on data. Employment rates of analysts project to grow 35 percent from 2022 to 2032, much faster than the average for all occupations. There are opportunities available for those who transition to data science career skills.
If your interest is piqued, let’s explore everything you need to know about changing careers to data science.
Reasons to change a career to data science
Not everyone can easily make the career change to a data scientist. It is a personal decision that requires significant thought (and a lot of motivation). Here are some of the most common reasons why you might want a data science career change:
Earning higher pay
The median annual wage for data scientists was $103,500 in May 2022. And Glassdoor depicts advanced data engineers who receive compensation of $183,000 per year (outside of possible other add-ons such as health benefits and stock). Such salary numbers are far above the average and can include considerable advantages beyond a standard engineer salary. For anyone looking at a data science career switch, compensation is a top reason.
Diverse opportunities
Information analysts have access to a wide range of job opportunities. You could focus on machine learning, or maybe decide to spend more time as a statistician. You could use your abilities for data mining, or work towards advanced status as an architect. You have a variety of possible career paths to explore.
In addition, data scientists operate within a range of industries. Finance, healthcare, and technology enterprises all need the support of skilled analysts. You can select from a diverse range of companies, each with unique projects.
Higher demand
At the moment, there is a talent gap. Companies cannot find and hire expert engineers at a fast enough rate. That also means that training practices are lagging behind demand. The gap may only expand as the employment growth continues at its current pace. The U.S. Bureau of Labor Statistics predicts total employment will grow by 11.5 million jobs over the 2016–26 decade, reaching 167.6 million jobs in 2026. We don’t know for how long, but at the moment, the future looks bright.
Increasing flexibility
After changes to work environments during the pandemic, data scientists have much more flexibility. Many engineers work remotely, with hybrid offices, or on flexible schedules. Outsourcing is common, as well as foreign country full-time working positions. That comfortable work-life balance may draw you towards a late-career switch to data science.
Creating better products
Data science is an intellectually stimulating field. Extracting information from raw data requires applicable knowledge that can satisfy any learner. The field covers numerous skill sets and project types. It combines elements of mathematics, statistics, computer science, and domain-specific knowledge. And bonus, many of the skills you learn are transferable to other occupations (don’t worry, your professional development won't be a waste).
In addition, a lot of your work can have an extended social impact. Many of the tools or applications you create will be used to build products that can change lives. From predicting diseases in healthcare to optimizing shipping routes, you can be part of fulfilling projects.
Growing professional skills
Outside of your technical skills, data science also offers plenty of opportunities to expand your abilities. You may find yourself working more on the business side as an entrepreneur. Or you may take on a leadership or managerial role in a larger firm. Data science adheres to continuous learning approaches, so at the very least, you will be able to expand the tools and frameworks you use throughout your career.
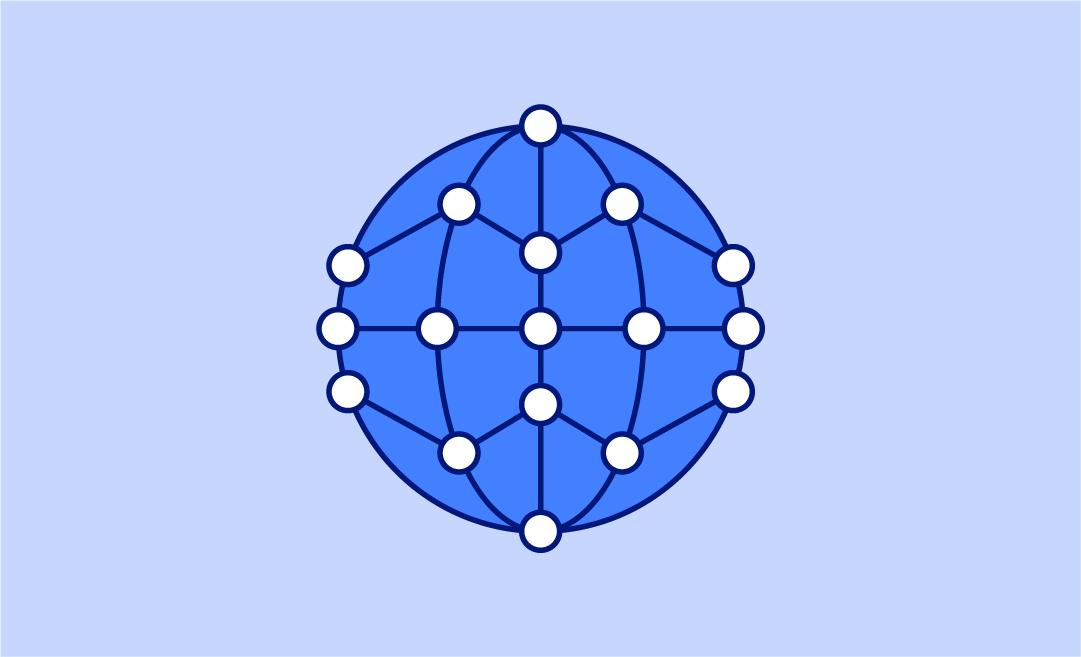
Select a data science role and evaluate your current skills
So you feel ready to switch careers to data science. How do you begin? How do you become an analyst?
At first, we suggest you assess your skills and determine the ideal path into the field. There are many different types of data scientists, and we have listed some of the most common below. Each role has its own responsibilities. Use the following list to help guide your research as you learn how to switch to a data science career. Ideally, you can select a possible data science career path that closely aligns with your current abilities and skill sets.
Data analyst
A data analyst works with data sets and helps clean and visualize that information. Extracted insights help companies with decision-making. As a result, you will need new skills in data visualization, statistical analysis, and data cleaning and wrangling. Access to a database usually occurs with Structured Query Language (SQL), so competency with SQL is a must.
Machine Learning (ML) engineer
An ML Ops engineer creates and implements all machine learning models. That can include algorithms for recommendation engines or predictive analysis. You also put the model itself into production, so programming ability (e.g. Python) is necessary. Of course, knowledge of machine learning, deep learning, neural networks, and modeling will be your main areas of focus.
Data engineer
Data engineers build out data structures. All components of the structure must match the use cases and designs of a data architect. Engineers ensure that all data collection processes occur efficiently.
That process is better known as Extract, Transform, and Load (ETL), where you retrieve data, process it, and store it in its correct repository. It is an area in the industry that demands lots of skill. Prepare yourself by learning data warehousing, database management, and pipeline management. In addition, skill with big data tools will be of help (e.g., Hadoop), along with advanced knowledge of a programming language. Learn more about the data engineering career path.
Looking to transition into the lucrative field of data engineering? Check out our roadmap on how to become a data engineer and kickstart your career.
Statistician
Statisticians use applied statistics for real-world applications. Ideally, data becomes a tool to create value for an enterprise. Statistical modeling helps with decision-making and the execution of current business activity.
Due to the strategy component, this role is often outsourced or provided as a consultation service. You will need extended diagnostic skills such as designing experiments and conducting statistical analysis (that includes Bayesian statistics). Knowledge of applicable software is also necessary (R, MATLAB, etc).
Business analyst
Business analyst job duties often overlap with those of a statistician or analyst, but business intelligence personnel have more reporting duties. Various stakeholders need a constant flow of digestible information in order to make accurate decisions, and BIs fulfill that need.
As a result, emphasis is placed on visualization. That includes an understanding of the IT language of data analysts, such as reporting and dashboard generation. You will also perform data querying and statistical interpretation. Technical ability with data display tools such as Tableau and Power BI are foundational.
Data architect
A more advanced and senior position, experienced engineers can progress toward a data architect role. Architects map out the overarching infrastructures. They also help guide all engineers in the execution of their plans. Without central coherence, all business operations will suffer from inefficiency.
The type of infrastructure skill you need will depend on your industry. For example, those who work in the cloud should know how to manage a cloud platform and serverless architecture. Other common attributes include architecture documentation, data governance, and database design. Gain knowledge about enterprise architecture frameworks such as TOGAF and Zachman.
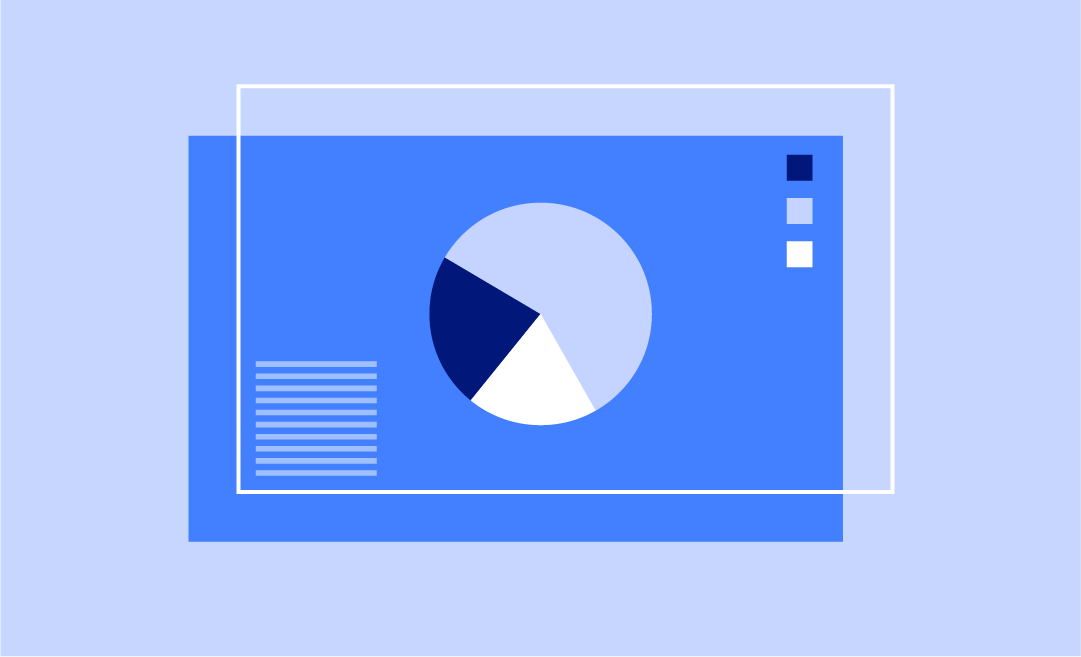
Transition tips for beginners
It’s one thing to make the jump into data science with a long work history as a software engineer. But starting as a beginner can include a different set of hurdles (and what you lack in experience can be made up with enthusiasm and curiosity). Follow these tips if you are transitioning to the field at the start of your career journey:
- Prepare your finances: The data science field is vast. You will need time and patience to develop your skills and build a robust portfolio. To that end, prepare your money ahead of time. Ensure your career choices are not hampered by financial constraints.
- Customize your resume: With limited demonstrable skills or work experience, it is important to highlight specific skills that apply to the data science job. Tailor your resume according to all application materials. In addition, take time to learn the best practices for technical tests and prepare for data science interview.
- Learn the fundamentals: As a beginner, domain-specific knowledge may seem necessary, but at this start of your career, recruiters for entry-level positions want you to have a healthy grasp of the basics (e.g., learn to answer the question “What is data science?”). Build a strong foundation in mathematics, statistics, and a programming language.
- Experiment with real data: Daily practice trumps theoretical knowledge. Test out your data manipulation skills with libraries like NumPy and Pandas. The more time you have to apply your skills and improve your data handling, the better. If possible, apply for internships or junior analyst roles.
- Seek mentorship: It's not what you know but who you know. Engage with other analysts in online communities, network with coworkers, and look for a mentor. Get feedback and first-hand career knowledge from those currently working in the field.
- Plan your professional development: If possible, map out your career trajectory. This will ensure that you focus on learning tools that directly apply to your desired industry. Take courses, boot camps, and if necessary, earn a formal degree. We have some data science material for self-study to get you started.
- Persistence: Dive into the deep end, and then stick with it! Often, your resume won’t meet all of the job description requirements, especially as you build up your skills. Still, it is better to put yourself through the hiring and review process. You may gain valuable insights from recruiters and can hone your skills. The start of your career may take time, so persistence here is a valuable trait.
Tips for transitioning to data science careers for software engineers
A late-stage career transition to data science will involve a different set of challenges than those experienced by new job market applicants. In many ways, you need to unlearn your current practices set at midlife and reorient yourself to the demands of the data science field. Here are some tips to help make the late career switch to data science a bit easier:
- Review the fundamentals: You might be an expert in your current field, but a shift into a brand new industry will have different basic skill requirements. Give yourself a solid foundation to build from by mastering the standard techniques.
- Advance your data manipulation skills: Unless you plan to start with an entry-level role, you will need ability with data exploration and data management. Take augmented classes for useful tools (e.g., Python). If applicable to the industry you plan to enter, place additional focus on machine learning algorithm creation.
- Study domain-specific knowledge: You already have a full resume with an extensive mid-career work history. Unfortunately, your past successes do not apply to your new field of data science. Remedy that problem by gaining domain-specific knowledge. For example, if you plan to become a data engineer, focus on ETL processes. If you want to work more in data viz, spend time learning storytelling. Chart out current skills to help identify any current talent gaps you may have. This is your chance to upskill!
- Earn relevant credentials: If you cannot get real-world experience working with the domain-specific knowledge you need to enter the field, start with credentialed courses. Amazon and Microsoft have numerous courses you can take to validate your abilities.
- Seek out networking opportunities: You likely have several work connections from previous career projects. Leverage those relationships to find entryways into the field. If that is not possible, join data science communities, work on collaborative projects, and visit conferences in order to meet with local data science professionals.
- Search for unconventional job opportunities: You do not have to follow the traditional corporate hiring structure in today's job market. Analysts can work in a variety of fashions. Consider alternative employment structures such as freelancing or consulting. You can also work remotely or operate as an outsourced worker. Even the type of enterprise you choose can affect your speed of transition, as there may be more demand in obscure industries such as crypto, ethical hacking, or sports analysis.
Conclusion
It is tough to transition into any career. But data science may be a more competitive employment market, as so many engineers are in search of new opportunities. The field of data science is growing exponentially — that demand is drawing in skilled workers from all across the globe. Plus, data science requires extensive skill across a wide domain of knowledge. The barriers to entry are higher than normal.
Still, with the correct roadmap and persistence, you can make the data science career transition. With the prepared skills, that's how to switch careers in data science. If you need help with your professional development, be sure to explore the education opportunities at the Anywhere Club. We have a designated learning hub, as well as a robust community of software engineers who can help you execute a data scientist career change.